Artificial intelligence leaders and researchers convened at Duke for a four-day symposium on responsible AI, placing discussions of AI ethics, alignment and labor market impacts in the limelight.
Among the symposium’s lineup of speakers Monday were Meta Chief AI Scientist Yann LeCun and Ronnie Chatterji, inaugural chief economist of OpenAI and Mark Burgess & Lisa Benson-Burgess distinguished professor of business and public policy.
The event, co-sponsored by the Society-Centered AI Initiative and other programs across health, engineering and sustainability, featured over 10 talks ranging academic disciplines and a Society-Centered AI Hackathon.
Yann LeCun
After receiving the symposium’s inaugural Society-Centered AI Distinguished Lecturer Award, LeCun shared his perspective on the state of artificial intelligence and where new development should be directed.
“First, what I want to say is that regardless of what your interest in AI is, at some point, we're going to need human-level AI systems,” he said. “They're going to assist us in our daily lives [and] be with us at all times.”
LeCun said he envisions a future where people can interact with AI similarly to how they interact with other humans. Still, he noted that there’s a challenge getting to that future.
“Machine learning sucks … in terms of sample efficiency, in terms of being able to acquire new skills quickly,” he said. “Machine learning is nowhere near the kind of capabilities that we observe, not just in humans, but in most animals.”
He added that researchers “have no idea” how to develop machines that function with comparable efficiency to humans, noting that large language models (LLMs) “spend the exact same amount of computation for every word or every token” that they produce.
So where should researchers look for solutions to these problems?
They should not look to training AI using videos instead of text, according to LeCun. Even though he believes that humanlike AI is not attainable when models are only trained to respond probabilistically with text data, training AI to understand and predict videos at the pixel level “just doesn't work.”
Instead, LeCun believes researchers should learn from babies and develop AI with the ability to observe and sense.
He recommended that researchers aiming to develop better models “forget about generative AI” and opt instead for joint-embedding predictive architecture. This method involves training AI models to understand and predict “representations,” or simplified states of the world. Models are trained to ignore irrelevant and unpredictable details and focus on how a given representation of the world will change if a specific action is taken.
He also emphasized the importance of making AI technology open source so that researchers outside of leading nations and corporations can advance the technology, advocating for a diversified “information diet.”
Ronnie Chatterji
Chatterji outlined his journey from joining the Fuqua School of Business faculty in 2006 to gaining tenure in 2014, where he cultivated his interest in the economics of innovation, entrepreneurship and technology.
In 2010, Chatterji joined the White House as a senior economist for the Council of Economic Advisers. In 2021, he began serving as the Department of Commerce’s chief economist in the Biden administration.
As the COVID-19 pandemic induced global supply chain shortages, Chatterji spoke of his realization that the United States’ dependence on Taiwan to manufacture semiconductors posed national security concerns. In response, he oversaw the implementation of the 2022 CHIPS and Science Act, which invested $280 billion in scientific research and manufacturing, including $52 billion in subsidies for the semiconductor industry.
This experience alerted Chatterji to the importance of investment in AI and other “deep technology” that require long-term infrastructure investments to cultivate major breakthroughs.
Narrowing the discussion to AI’s potential labor market impact, Chatterji shared a wide range of forecasts by “credible economists” about annual AI-driven productivity growth in the U.S. economy, with estimates ranging from 0.06% to between 7% and 18%. To Chatterji, these values reflect significant but divergent estimates about the impact of AI.
Get The Chronicle straight to your inbox
Sign up for our weekly newsletter. Cancel at any time.
This is consistent with a trend in “technological history,” where there is “divergence before convergence” in predictions about emerging technologies, he added. For AI, Chatterji believes this divergence is rooted in competing views of LLMs either as a “helpful editor and summarizer” or agents that can “build and scale.” He added that adoption of new technologies — and subsequent productivity gains — differs by geography and industry.
“This is, to me, the one blind spot for a lot of people who are thinking purely in technological terms,” Chatterji said. “They completely underestimate, often, how long it takes organizations to change and how resistant people in organizations are to change.”
As evidence of AI’s uniquely rapid growth, he shared that modern AI systems are surpassing performance benchmarks at an increasing rate. Chatterji pointed out that OpenAI’s ChatGPT had over 100 million users within two months of its launch, a pace of adoption he described as “pretty amazing” and lacking precedent.
He also conveyed enthusiasm about AI use in education, explaining that AI tools can complement teachers by offering “different ways of learning the same concept” and “tailoring” teaching methods to specific students.
According to Chatterji, the OpenAI economic research team’s current work includes engaging with the research community and creating an “innovative AI tool that enhances qualitative survey responses,” in addition to measuring the impact of AI within a statewide higher education system, a multinational corporation and a small country.
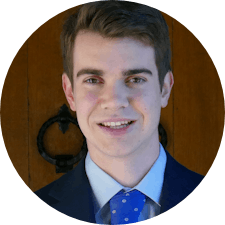
Michael Austin is a Trinity junior and managing editor of The Chronicle's 120th volume.
Max Tendler is a Trinity first-year and a staff reporter for the news department.