We’re not going to label Twitter as “Chaotic Good” or “Chaotic Evil,” because let’s be honest, it’s purely “Chaotic Chaotic.” As we write this article, “adderall” is trending worldwide under the category “politics.” It also seems like someone’s looking for a missing parakeet in the Boston area. Have YOU seen Harry?
Twitter is a funhouse mirror of our world. It’s one of the few corners of the internet where you can see updates from your local journalists, celebrity chefs, self-proclaimed anarchists, grassroots political activists, and your neighbor’s dog account all in the same place. It’s just great!
While Twitter, the home of the hashtag, is not the largest social network in town, its influence on culture and events has been pervasive. Movements including The Arab Spring, #MeToo, and #BlackLivesMatter have used the platform to disseminate information. Celebrity gossip and memes often originate on the site. Twitter accounts have become a staple of every newsroom, given that 71% of Twitter users say that they get their news from the platform.
With a cult-like following, the platform often feels like a laissez-faire free-for-all, serving as both a productive news-bearer and an escapist haven. But we cannot forget that Twitter is actually an intentionally-designed space. Every feature, like buttons or threads, has been crafted by a team of designers and engineers, who each bring their own backgrounds and perspectives to the table.
If you watched even just the trailer for Netflix’s documentary “The Social Dilemma,” you know that the websites we interact with each day are hyper personalized thanks to algorithms. Simply, algorithms are the processes or sets of rules that dictate how social media platforms operate.
Like in the case with TikTok, algorithms sorts users based on preferences and characteristics in order to provide them with “relevant” information. While personalization can be helpful, it can also backfire. Users can become entrapped in “echo chambers,” “filter bubbles,” or, as one professor puts it, “algorithmic content hell.”
But Twitter takes that one step further: not only does Twitter’s algorithm warp the content of your feed, it also decides which tweets you see first, what trends you might be interested in, and how you see photos that are posted to the platform.
So when the Twitter algorithm decides to resize a photo and hide non-white faces, it’s not just a fluke. It’s a major problem.
Recently, Colin Madland tweeted about an issue with Zoom’s facial recognition algorithm. His colleague was having repeated difficulty using Zoom virtual backgrounds and sought his help. Madland quickly determined that it was not a technical misdoing on his colleague’s end, but rather, a problem with Zoom: the algorithm removed the face of his Black colleague and replaced it with a pale desktop globe.
Yet, the problem didn’t end there. When Madland tweeted the screenshots of him (a white man) interacting with his colleague (a Black man), the Twitter algorithm resized the photos and cropped the colleague out of the photos entirely.
This occurrence was not unique to Madland’s Twitter account. Thousands of people began to run their own tests, including us. In our little experiment, we created an image that includes our headshots placed on opposite sides. We then flipped the photo to create a second image.
When we created a “test” tweet with the two images included--without any blurring-- the algorithm automatically resized both of the images to only show the headshot for Jess, who is white, on the mobile version of Twitter.
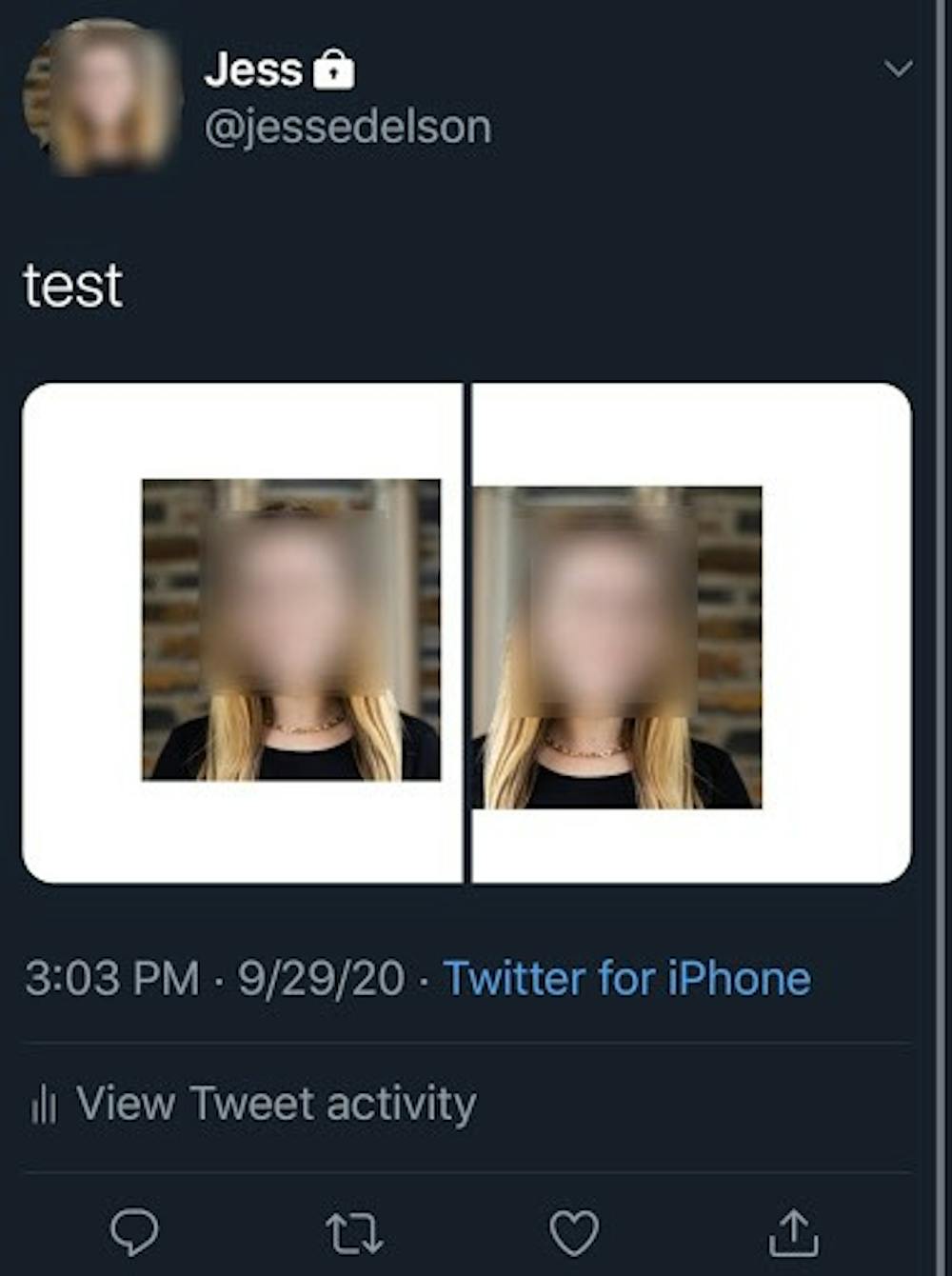
If somebody were casually scrolling through Twitter and came across this tweet, they would not even notice that Niharika, who is Asian, was in either of the photos. Even when the images were both flipped, the image preview ends up being the same. This is clearly a flaw in Twitter’s algorithm.
Dantley Davis, chief design officer at Twitter, would have you think that Colin Madland’s facial hair and dark-colored glasses are “affecting the model” due to the high contrast. So does Twitter’s facial recognition algorithm identify facial hair rather than, you know, actual faces? If a pair of glasses and a beard are going to mess up the algorithm, maybe they should consider building an algorithm that resizes images without considering the image content.
Even if you chalk that last one up to an accident, how would Twitter explain the discrepancy between photos of Mitch McConnell and Barack Obama? They’re both well-known and frequently-photographed, so there should be no dearth of possible training data. As politicians, they even have special portraits so you don’t have to worry about objects in the background.
It’s ridiculous. It’s blatantly biased. And this pervasive bias is not a one-off “error.” It’s built into the core of Twitter’s system.
To Twitter’s credit, the company’s communications department said they didn’t find any racial or gender bias when they originally tested the model. But how does Twitter actually find evidence of racial and gender bias? How did they miss this key issue with the mobile image preview, which has since been duplicated by many other users?
Twitter’s CTO, Parag Agrawal, wrote “Love this public, open, and rigorous test—and eager to learn from this.”
That doesn’t acknowledge the impact this fundamentally flawed model has had on users, who may not have realized how their images were being cropped by the platform. When artists, political activists, and others on the platform are trying to amplify their message, they might not realize that a whole part of their photos are being erased by Twitter.
This “public, open, and rigorous test” that Agrawal is excited about does not absolve Twitter of the responsibility of conducting rigorous testing on their end to weed out racial and gender bias from their machine learning models. It’s great that tech-savvy users can catch these errors in practice, but the burden shouldn’t be on users to find the deeply baked-in bias in Twitter’s neural networks.
Twitter, of course, is not the only company with a fundamentally flawed facial recognition algorithm—in a review of major facial recognition tools in industry, researchers found that this technology consistently underperforms for women and people of color, with women of color doubly impacted by algorithms that haven’t been rigorously tested for bias. And several of the companies that develop facial recognition tools have hit pause on those programs, in light of how facial recognition has perpetuated racial biases in policing.
It’s a step in the right direction. Twitter’s communications team has acknowledged that “it’s clear that we’ve got more analysis to do.” Given that their users interact with the product frequently, sometimes scrolling through feeds multiple times in a day, Twitter owes it to all of us users to be completely transparent as they move through their analysis.
And that doesn’t mean just putting indecipherable code on a website hidden behind complex legal jargon. Twitter needs to publish their analysis in a way that can be understood by the average user, with updates included at the top of users’ feeds.
Twitter’s review of the training datasets should not mean throwing a bunch of statistics on a report—Twitter needs to actively review their training datasets, at least on an annual basis, to understand who is underrepresented in the datasets and adjust the datasets accordingly. If photos of underrepresented groups, like women and people of color, are unclear, new photos need to be added and the facial recognition model must be retrained.
This isn’t just a problem with photos in a database-—we have to recognize that the lack of diversity of our technology workforce is inevitably reflected in the technologies we all use. If the identities of a technology’s creators do not reflect those of its users, we can’t expect meaningful change in the algorithms that drive our digital interactions.
If Twitter had a more diverse team, perhaps the major issues caught in the “public, open, and rigorous test” would have been identified earlier in the product development process. Maybe then, users wouldn’t have to do Twitter’s work of finding obvious, shocking flaws in the algorithms after the fact.
We urge Twitter, and other companies utilizing facial detection and recognition algorithms, to frequently examine their models, bolster diversity, and provide transparency in how they are reviewing and revising their models and their training data.
As the adage goes, garbage in will equal garbage out in our algorithms. So, Twitter, why not harness that chaotic energy to prevent the next dumpster fire?
Jessica Edelson and Niharika Vattikonda are Trinity juniors. Their column, “on tech,” runs on alternate Thursdays.
Want us to break down a technology topic you’re interested in? Email us at jre29@duke.edu and nv54@duke.edu.
Get The Chronicle straight to your inbox
Signup for our weekly newsletter. Cancel at any time.